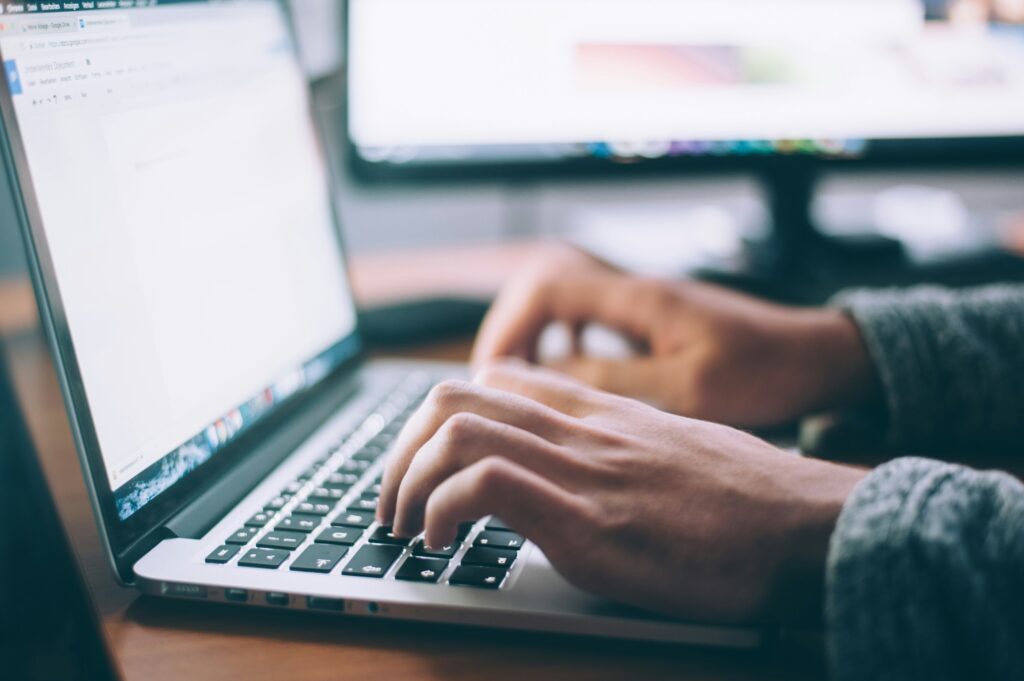
The Tools Competition strives to provide value to all competitors, regardless of whether or not they progress in the competition. The competition is grateful for your time, and eager to share high-level feedback and resources to support all competitors to advance their ideas to improve teaching and learning.
Feedback on Accelerating K-12 Learning and Enhancing Post-Secondary Learning abstracts that did not advance to Phase II:
Each year, the Tools Competition receives an increasingly competitive pool of abstracts. The evaluation process is rigorous, prioritizing potential for impact and abstracts supported by evidence. As the commitment required in subsequent phases is significant, only highly aligned abstracts with strong likelihood to perform well in future phases of the competition advanced to Phase II. This approach also reflects feedback from competitors, who have shared preference for a smaller, more competitive group to advance to Phase II given the rigorous requirements.
Based on our review of all Phase I submissions, we’ve compiled some feedback to highlight common reasons why submissions did not advance to Phase II:
- The tool did not align with competition tracks’ objectives and/or target audiences: The competition only advanced abstracts that meet the track’s specific objectives, serve the target audience, and meet the eligibility requirements for a given track. Evaluators considered alignment around eligibility requirements, including target geographies, age groups, and/or audiences. In some cases, the competition shifted strong abstracts to another track if not a fit in its original track. For more information on specific eligibility criteria for each track, review each track page on our website.
As a result, many thoughtful, compelling abstracts did not advance because they solved a problem that is not specifically targeted in one of this year’s competition tracks, sought to serve a different population, or did not demonstrate strong alignment to student or adult learning outcomes. Here are a few specific examples: (1) In the Accelerating Learning track, we received some submissions focused solely on early childhood education. While this is an undoubtedly important group of learners, this track requires that the tool serves learners in K-12. Tools that served both early childhood learners and learners in K-12, however, were eligible to advance. Or, (2) In the Post-Secondary Learning track, only competitors in the United States are eligible to submit. As a result, though the overall competition is global, we did not advance high-quality abstracts in this track if they were not from competitors in the United States.
- The abstract did not clarify what was ‘new,’ and/or the innovation and novelty was unclear: The competition seeks to fund innovative ideas through new tools or enhancements to existing tools, with high priority given to tools that demonstrate uniqueness or highlight advantages over similar products in the market. Given this, some competitors did not advance if their abstracts broadly described an existing tool and sought additional funding to support operations or expansion efforts. The strongest abstracts represented exceptionally unique ideas, or, in categories with many similar concepts–such as learning management systems, math learning tools, student feedback tools, or lesson plan generators–effectively highlighted their competitive edge, existing uptake, or evidence supporting their tool.
- The abstract was not explicit in its intent to support learning engineering: Learning engineering underpins the objectives of the competition. The competition seeks tools with the potential to generate robust learning data that can answer important research questions, and teams dedicated to rapid experimentation and continuous improvement. The most compelling responses outlined the specific learning data their tool would collect, the questions this data could address, the role of the data in continuous improvement, and well-defined intention to support external research. Conversely, some responses focused solely on how data might be used to aid in tool improvement, the general importance of learning engineering, or a single study planned of the proposed tool – these were likely scored as incomplete.
- There were major risks or barriers to scale: Some tools face inherent challenges in scaling, whether due to a saturated market, the need for physical components, or other factors. In these cases, strong abstracts outlined potential strategies to mitigate these barriers. In contrast, some competitors did not advance because their abstracts did not adequately address significant scale concerns. The strongest responses may have highlighted what makes their tool inherently scalable as well as existing partnerships or recognition that can support scale, where applicable.
- There was minimal evidence to support the tool or approach. Some abstracts described exciting tools but lacked the evidence or compelling detail to support the claims, and demonstrate why the tool will be successful or why the approach is effective. Similarly, some competitors with existing tools did not provide insights into the tool’s traction, how it has been received by users, or evidence supporting its impact on learner outcomes. This detail often makes the difference between a proposal that stands out and one that is not successful. Certainly, in a short abstract, proposals aren’t expected to detail all relevant research. Depending on the phase of development, competitors may briefly point to specific evidence such as the need for the tool or approach, adherence to frameworks or content standards, user research findings, partnerships, recognition received, or the efficacy of the tool itself. Although Phase I abstracts are concise, the strongest submissions still managed to highlight this information, even if briefly.
- Less competitive compared to other proposals, if a team submitted multiple proposals. Many competitors submit multiple different ideas for tools in Phase I of the competition. Some like to take this approach as a way to pressure-test their ideas with an external review audience. Given the rigorous requirements in Phase II, many competitors ask organizers to help prioritize which of their Phase I abstracts would be most competitive. In most cases where teams submitted multiple compelling abstracts, competition organizers selected the strongest or most aligned submission from each team to advance. If you have a question about the proposal that was selected to advance, reach out to our team.
- The abstract lacked detail, focus, or included significant errors: Phase I abstracts are short, but the competition looks for thoughtful ideas that address all of the topics outlined in the submission form. Some abstracts presented broad, all-encompassing ideas that were described at a high-level, raising questions about the feasibility, how all the elements would be effectively executed, and what the overall objective of the tool or proposal was. In contrast, the most compelling abstracts had a clear focus, described the specific features they were proposing, and provided sufficient detail to provide a clear picture of the user experience and how the tool would function. Further, abstracts that lacked sufficient detail to clearly explain the idea, despite having additional space to do so, did not advance. Finally, abstracts with numerous errors, such as incomplete sentences or significant typos, did not advance, as these issues hindered our ability to fully understand the ideas presented.
Feedback on Dataset Prize abstracts that did not advance to Phase II:
The Tools Competition was thrilled to introduce a new opportunity for the Dataset Prize this competition cycle. This new track had hundreds of submissions, many with significant potential for impact in education technology. For those not advancing to the next phase, here are some common points of feedback:
- The abstract did not focus on teaching and learning: This track prioritized datasets with a clear focus on the experiences of learners or teachers in an educational environment (physical or digital), where the dataset was designed to drive the development of technologies that address their urgent needs. While some datasets were novel and valuable for research or innovation, they fell outside of the core domain of teaching and learning.
- The abstract did not demonstrate a clear application for AI innovation: Abstracts were considered for the integration of advanced AI and machine learning methods. Some datasets, while useful for research purposes like informing educational policy or practice, did not demonstrate a clear application for training sophisticated AI models or serving digital learning platforms. Our focus was on datasets that could directly enable advanced AI model training and support use cases for data scientists and developers.
- Details on Criteria: The Dataset Prize featured different categories of criteria. Here are some overall reflections on how proposals may have failed to meet a dimension of these criteria:
-
- Size: The proposal failed to demonstrate that the dataset is sufficiently large to train AI models capable of achieving or exceeding state-of-the-art performance on specific tasks. Most datasets for this purpose need at least several thousand data points or more than 500 learners/observations.
- Novelty: The proposal may not have clearly shown that the dataset offers unique features, such as webcam-based eye tracking, or addresses underexplored educational challenges. For instance, due to the large amount of online tutoring and learning tools, there is a lot of data available on student engagement with these tools. Additionally, some abstracts described datasets that were similar to benchmarks The Learning Agency or its partners have funded. Proposals needed to highlight the unique nature of their data such as new approaches, large size, demographics, use cases, etc.
- Equity: The dataset may have lacked robust learner metadata, such as grade, race, or special needs status, and does not ensure adequate representation of historically marginalized groups. In some proposals, the intended population was not certain or it was hard to tell if metadata was included. This limits the dataset’s ability to enable AI customization for diverse learners and to address bias effectively.
- Research Utility: The dataset may not convincingly demonstrate its potential to advance research in areas where AI has yet to reach or exceed human performance. This aspect correlates closely to dimensions of novelty and versatility. Essentially, data needs to illustrate how this could push forward or improve the state of the art.
- Versatility: Some datasets may have been too constrained in the data included or lacked detail. The Dataset Prize was particularly interested in supporting datasets that could be used in a variety of R&D use cases and include rich contextual or multimodal data.
- Generalizability: The dataset may have contained highly specific or niche information that, while novel, could not be readily generalized to other learning environments. This limits its broader applicability and reduces its value for advancing AI in education. While there is great value in studying smaller communities or more niche topics, the track this year was particularly interested in datasets that could be used for learning engineering outcomes with broad implications and impact.
- Team Readiness: In order to prepare data for learning engineering, transformation and preparation of the data are needed. To understand and translate the needs of learning engineering to the preparation of data, the team needed to demonstrate a strong command of their data and an understanding of how their data can be used. Proposals with deep explanations of the data, its potential use cases, and furthermore align this with their team’s expertise or skills were the strongest.
Resources and opportunities to stay engaged:
The competition is eager to support all competitors. We are humbled to see the amazing expertise, passion, and impact among all competitors and are dedicated to providing resources to support this community.
Organizers will continue to build additional resources to support Tools Competition competitors and the learning engineering community overall. Initial resources are available below.
- Connect and share ideas with the community. Competition organizers are eager to foster collaboration and community among the learning engineering community. Specific opportunities include:
- Join the Learning Engineering Google Group. The Learning Engineering Google Group is a global community of researchers, innovators, and practitioners committed to advancing the field of learning engineering. This is a great place to connect with others and get feedback on your ideas. Members and competition organizers also regularly post interesting articles, share upcoming events, and circulate additional funding opportunities. While this is not an official Tools channel, it is moderated by our colleagues at The Learning Agency.
- Join a monthly virtual networking session to connect with others in the learning engineering community. The Learning Agency hosts networking sessions on the first Wednesday of the month at 1pm Eastern Standard Time for individuals in the learning engineering community—broader than just the Tools Competition. During each session, participants break into smaller groups to share ideas, receive feedback, and learn from one another. Register here for the next session on Wednesday, December 4th, 2024.
- Explore other capacity building opportunities. We have identified a few helpful resources below. Note that the Tools Competition is not officially partnered with these groups, but they represent a few leading organizations, including those that have worked with Tools competitors in the past.
- For US-based organizations, the Community Funding Accelerator (CFA) is another excellent resource. CFA helps underserved communities win federal grants as well as tracks available federal grant opportunities for a larger community. Check out their federal grants tracker for opportunities that may be relevant to your work.
- The eLab Fellows Program brings together a global community of entrepreneurs leveraging tech for education across K-12, higher ed, and workforce development. Fellows learn tools and tactics in leadership, culture building, strategic decision-making, and more. Applications are accepted on a rolling basis.
- International Centre for EdTech Impact. Offers services, free resources, and select pro-bono advisory support from learning scientists focused on evidence-based development of learning technologies.
- Edtech Recharge. Offers services for capacity building, research, and impact; as well as numerous free resources, webinars, and templates supporting strong proposal development.
- RYE Consulting. Offers services and workshops, technical assistance, and regular free webinars focused on building and scaling K-12 edtech businesses, with expertise on US markets.
- Promise Venture Studio. Runs an accelerator program, collaborative, demo series, and other resource, community, and advisory services for teams focused on early childhood education.